Introduction
There are many compelling theoretical reasons why Bayesian statistical methodology is superior to classical, “frequentist” methods. Under weak conditions, Bayesian estimates are “admissible”, to use a specialized decision-theoretic term. Bayesian methods base inference only on the data actually observed, rather than on the probability of data you might otherwise have seen, but didn’t (the basis for p-values). This makes the study designer’s intentions critical for calculating a p-value, which can lead to all manner of absurdities.
This begs the question: what are aspects of a problem that make a Bayesian approach well-suited for it? And what is it about the Bayesian approach that makes it so? And–most challenging–can I make an argument that a non-statistician would find useful?
I need to give a little technical background, and then I’ll offer an example.
Meet the likelihood function
Definition
We observe a data-generating process governed by unknown parameters collectively named \(\theta\). We refer to the data collectively as \(x\).
If we are considering data that we might get in the future from a process governed by particular values of \(\theta\), we are applying probability. We think about probability distributions, cumulative distribution functions, densities, etc. On the other hand, if we have already observed data and we are now wondering what we can learn about parameters , we are in the statistical estimation and inference world.
- Here’s a very powerful concept: imagine writing down a mathematical expression for observed data \(x\) given parameters \(\theta\). This is based on prospective thinking. However, if we then consider \(x\) to be fixed, we have a function of variable \(\theta\). This is the “likelihood function”.
- If observations are independent, this function is a product of each individual observation’s likelihood.
- Applying the logarithm turns multiplication into addition, so the product of contributions becomes a sum of contributions to the “log-likelihood” function.
- If \(\theta\) are continuous, the (log-) likelihood function is a curve or surface over all possible \(\theta\) values, some getting a higher likelihood than others. We think of this as the vehicle by which data argue for certain \(\theta\) values over others.
If likelihood gives weight to values of \(\theta\) that indicate a model that’s more or less consistent with observed data–i.e., the model fits–then the \(\theta\) value that maximizes the likelihood could be a good statistical estimate. And it usually is: this is the maximum likelihood estimate (MLE); this approach has been massively successful.
Likelihood surface and weight of evidence
You might also ask whether there are other \(\theta\) values that give likelihood close to the maximum. Is the likelihood surface unimodal? If so, how quickly do values drop off as we move away from the maximizing point? This provides information about the precision of the estimate.
In fact, you could imagine developing an entire framework for assessing evidence and making estimates using only likelihood and eschewing p-values. Indeed, Richard Royall has done just this.
Like Royall’s construction, Bayesian methodology also builds an entire inference framework on likelihood, but with an additional ingredient: a “prior” probability distribution reflecting the state of knowledge about \(\theta\) prior to seeing the recent data. This is not a sampling-based probability, or rate; it is probability representing subjective belief.
Bayesian estimate
Note that we have two objects that span the space of all possible \(\theta\) values and provide a weighting of such values: the prior and the likelihood. The Bayesian approach proceeds by taking the product of the two. If a true distribution (for \(\theta\)) is needed, we only need to find the denominator for this product that makes it integrate to 1.0. Often this is not required, and in fact in most non-trivial situations we come to understand this distribution by sampling from it.
There is another very interesting wrinkle: for a given value of \(\theta\), we have a sampling probability distribution for future \(x\). Now, instead of just one \(\theta\), we have a weighting over all possible \(\theta\), in light of past data \(x\). We can develop a “predictive” distribution for future data, based on past data, by doing the following:
- Generate random sampling draws of \(\theta\) values from the posterior distribution;
- Given the \(\theta\) values, generate random draws of \(x\) values.
What makes the Bayesian approach work well?
What features of Bayesian methodology make it work well, and for what sort of problems? In my experience, they are these:
- Bayesian estimates (and inference) are stable and usually well-behaved in situations where frequentist estimates are not.
- This is due to “shrinkage” estimation which generally helps more than it hurts.
- Inference in complicated scenarios is usually not hard. This is especially valuable when there are many parameters, and we’re entertaining hypotheses about relationships among them. Practically, most Bayesian applications nowadays wind up with samples from the joint posterior distribution, and complicated assessments become a matter of counting, or density estimation.
- The predictive distribution is powerful for prospective thinking; the frequentist world has nothing quite like it, though it tries.
- An specification interval or normal range based on a Bayesian predictive distribution is more reasonable and intuitively appealing than a tolerance interval–but that’s a topic for another day.
Shrinkage, a hedge against gullibility
A note on stability: While maximum likelihood estimation usually works well, it can exhibit a “gullible” quality with sparse data or small data sets. Take a pathological example: estimate a binomial rate \(p\) based on only one observed data point \(x\); suppose the observed \(x\)
is a success. No reasonable person would give credence to a parameter estimate based on only one observation, but the MLE mathematically exists, and its value is 1.0.
A Bayesian posterior mean also exists, and depends on the prior distribution; if we adopt a uniform distribution from zero to 1.0–intuitively appealing, and also analytically tractable–we find that the posterior distribution has the Beta(2, 1) distribution. This distribution has mean 2/3.
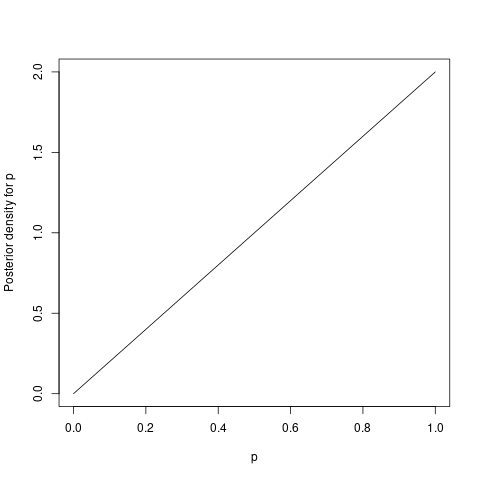
We still shouldn’t give much credence to this value, but note that the methodology is “hedging”, pulling the estimate towards the prior mean, 0.5. The figure above indicates that the single a priori most likely value for \(p\) is 1.0, yet the posterior distribution remains quite diffuse, hence the expected value of the distribution is substantially lower than 1.0. We refer to this as “shrinkage”, and it is inherent in calculating a weighted average over all possible values of \(\theta\) (in this case all values of \(p\)) rather than finding a single maximizing value.
In fact, the lower tail containing 5% probability can be obtained from a simple probability lookup from the beta distribution, and it is 0.22. Hence a Bayesian analog to a 95% confidence interval is (0.22, 1.0), appropriately very wide.
Furthermore, we can structure how the shrinkage happens, if not its amount. Do we shrink towards zero a null value, such as zero (for model coefficients) or 0.5 (for probabilities)? If we have multiple parameters, do they all shrink towards a null value, or towards a central value that is not determined a priori? The latter can be accomplished with a hierarchical prior, where parameters of the prior distribution are drawn from a “parent” distribution.
An example
Years ago I was pulled into a project to develop QC specifications for batches of incoming material. Since the project was new, there were not many batches available, fewer than 20. Moreover, the manufacturer had changed their process midway through the project, and it was not known whether this change had influenced the subsequent batches. We needed specifications on roughly 20 metrics, so we needed to have an analytical process that was widely applicable.
For each metric, we have a mean \(\mu_2\) pertaining to batches after the change, and \(\mu_1\) pertaining to before the change. Naturally, for projecting into the future, we’re interested in \(\mu_2\). It’s possible that \(\mu_2 = \mu_1\). Similarly, we have \(\sigma_2\) and \(\sigma_1\), and it’s possible that \(\sigma_2 = \sigma_1\). We deemed it unlikely that variance would change appreciably, and elected to assume a constant \(\sigma\) barring strong evidence to the contrary, although we could have set up a shrinkage framework for two variances. In either case, the pre-change batches provide useful information about variability, and so are not discarded.
Note that if we elected to allow different variances, the frequentist would probably cast out the pre-change data as irrelevant to predicting future data, leaving a painfully small data set with which to set important specifications. A Bayesian can adopt a hierarchical prior in which the twgo variances are shrunk towards a common value, by a degree that is determined in light of the observed data. This isn’t entirely fair to frequentists; it is possible to contrive some sort of shrinkage estimation without using Bayesian methods. But determining the degree of shrinkage–and rationalizing that choice–remains a weak link.
I set up a Bayesian ANOVA framework with common variance but a hierarchical prior on the means, to allow the pre-change mean to differ from the post-change mean by degree determined by the data. I then drew samples from the predictive distribution for the post-change group, and calculated quantiles from this sample.
Some observations from the example
A few comments:
- By allowing means to differ, I added a parameter.
- For a frequentist, this would mean adding another degree of freedom to a search process, potentially increasing gullibility and raising the possibility of overfitting.
- It also requires a more complex workflow: one must decide whether \(\mu_2 = \mu_1\), then act accordingly. The statistical reliability of the result is now a bit compromised because the final inference doesn’t comprehend this decision-making step.
- Because the Bayesian estimate is a weighted average over all possible parameter values, the consequence of a more-complex model is not overfitting but rather a more diffuse posterior distribution with increased shrinkage of parameter estimates. We don’t get something for nothing, but with very small data sets, Bayesian estimates “fail safer” than frequentist methods.
- In some cases analysts applying Bayesian methods to fit single-hidden-layer neural nets do not carry out the cross-validation that is de rigueur for standard machine learning. If you’re an analyst, let that sink in. Bayesian neural nets do not smoothing parameters to be carefully optimized, and they do not overfit, even with many (or too many) parameters.
- For a frequentist, this would mean adding another degree of freedom to a search process, potentially increasing gullibility and raising the possibility of overfitting.
- The case above, involving a production change which may or may not induce process change, is an example of ancillary factors complicating primary questions of interest.
- This comes up often and is an enormous time suck on our economy, especially when the analysis is going to be reviewed by regulatory authorities. The general pattern is this: We want to know if \(A\) is equivalent to \(B\), but we have other factors \(C\) at work (production change, different reagent lots, different labs, etc.) which may or may not have an impact. With frequentist methods, we first make a decision concerning whether to use a large model (accommodating differences in \(C\)) or a simpler model (assuming no effect of \(C\)). Sometimes the conclusion depends on the model, and then what?
- My proposal: if in doubt, use a hierarchical Bayesian model that allows for ancillary difference in a way that induces data-dependent shrinkage, such that the predictive distribution leaves 99% of future cases within specification.
- This is an example of a computationally complex application that yields conceptual simplicity and a simpler analysis workflow.
Some cautionary notes
Hopefully this essay provides some intuition into what Bayesian statistics does well, and what sorts of problems are amenable. But in fairness, I should also note some challenging aspects:
- Bayesian methods use the likelihood function. Therefore a specific probability model is absolutely required. If that model doesn’t fit, the system can’t be expected to work well.
- Similarly, Bayesian methods don’t make any provision for handling outliers. To do so, the model must be expanded explicitly. There have been some very effective efforts in this direction but they have not become widely used.
- Therefore you’ll need to check data for outliers. If you find them and they are potentially influential, you’ll need to exclude them or winsorize them so they don’t corrupt the model. And then discuss the exclusion and its implications.
- The problems that benefit the most from shrinkage are exactly the problems that are challenging for the sampling methods that Bayesians have been using for years. Shrinkage helps when there are many parameters, which are collectively important but for which no one individual plays a distinctly strong role. In this case, the likelihood indicates correlation among the parameters. Fortunately sampling technology has advanced, such as with Stan, though Stan allows only continuous parameters.
- Bayesian methods require a prior distribution. What if you perform a sensitivity analysis and it makes a difference to the conclusion?
The last point deserves a little discussion. This is perhaps the criticism of Bayesian methods that is most common: subjectivity plays a role.
Practically, the predominant practice currently is to use very vague prior distributions, so that when the data set is large enough, details of the prior distribution become practically irrelevant. Having the structure of the Bayesian approach can still provide value, particularly with a hierarchical prior that imposes the desired form of shrinkage.
What if one has a critical question, yet is limited to a small data set, and the prior makes a difference? Then you don’t have enough data for the data alone to determine the conclusion. On the positive side, at least you know this is the case. If you must make a decision, then know that your expert opinion is going to play a role, so get the best experts you can and elicit their opinion carefully (i.e., fit a prior).
If a prior sensitivity analysis demonstrates that data is not decisive, and a frequentist analysis indicates that data is, which should you believe? I think using the Bayesian approach in this case would lead to better long-term decisions.
Summary
If you’re an analyst or someone working with an analyst, your problem could be particularly amenable to a Bayesian approach if it has any of the following characteristics:
- You have limited data, but the inference questions are critical.
- You have many parameters, and you’re particularly interested in only some of them.
- You’re particularly interested in future data, such as specifying a normal range or a specification range.
Leave a Reply